If you want to see how this can apply to your business, keep on reading.
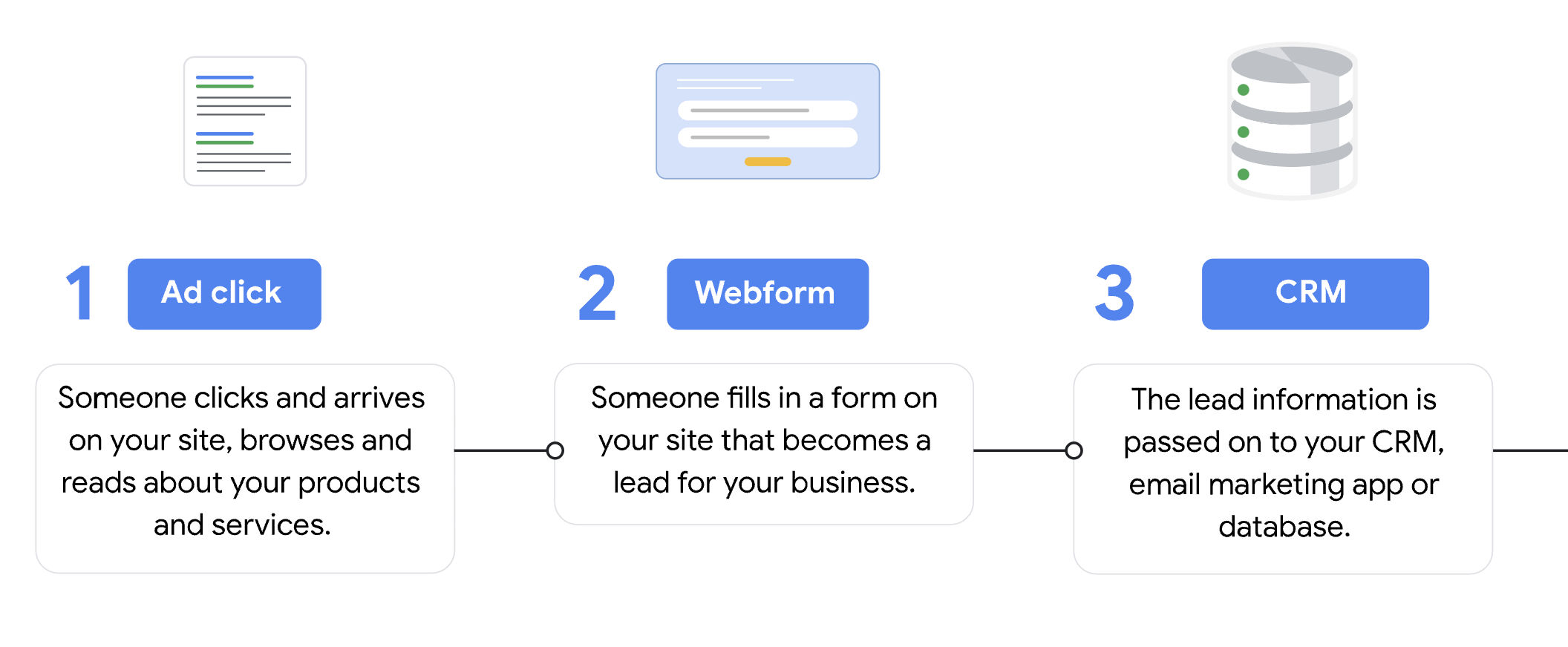
Google Ads launched Enhanced Conversions for Leads as an alternative to Google Click ID-based offline conversion tracking method. It may be easier to adopt for advertisers since this can be set up directly from your Google Ads account, so this data could mean more efficient conversion tracking.
Have you ever used offline conversion tracking? If so, how tedious was the setup experience?
How does it work?
When users fill out a form on your site, you’ll receive data including email, name, address, and phone number. This data is captured in your conversion tracking tags and then sent to Google. When it converts, Google matches the information received to the ad that caused the lead, providing a more complete picture of the user journey.
The issue with the existing Google Click
Offline conversion tracking can help Google get the idea of the value of leads, prioritizing the most valuable ones. Even so, this method has not been widely adopted due to the difficulty of implementation.
However, the new Enhanced Conversions with Leads uses the information you’ve already captured such as email addresses, so there is no need to modify CRM systems or invest more time figuring out complex integrations between CRM and Google Ads. This is why Enhanced Conversion with Leads might be a convenient solution for many advertisers.
Did you encounter this issue? Let us know in the comments below!
Where can you find it?
Enhanced Conversions for Leads can be found in Google Tag Manager if you have Google Ads conversion tracking set up. Also, it can be configured using the global site tag on your page if you already have conversion tracking implemented.
We will continue keeping tabs on this new method and see if it is a more convenient solution. Stay tuned!
About Quantikal
Quantikal is a data-driven performance marketing agency based in Buenos Aires, Argentina. We help clients scale their business through profitable digital ad spending by constantly obsessing over clients’ key metrics. Quantikal’s experience working in diverse online companies in marketing roles including media buying allows us to provide solutions in the ever-demanding increase of marketing performance, doing more with less thus becoming a strategic partner.